Business Brief
Improve Cost-Effectiveness Analysis and Budget Impact Modeling Using Literature Review Automation SoftwareThere is a substantial proliferation of published cost-effectiveness analyses and budget impact assessments, resulting in an increase in systematic literature reviews (SLRs). Concurrently, advances in automated literature screening, classification and data management are enabling researchers and decision makers to interpret these studies faster and more accurately and subsequently apply the results to clinical practices.
Increasingly, healthcare decisions are based on intersecting data of clinical evidence and value, including cost-effectiveness analysis (CEA) and budget impact modeling (BIM). Many CEA and BIM assessments utilize systematic literature reviews and meta-analysis to estimate economic, patient-reported, and clinical consequences. SLRs are used to synthesize the multitude of cost studies for use of in-value assessments. These evaluations guide health economics and outcomes research (HEOR) professionals and other decisionmakers to deliver better clinical care, improve healthcare policy, and manage costs.
Cost-based studies are noted for their heterogeneity of methods, outcomes, and perspectives. This makes them particularly challenging to interpret. HEOR researchers are seeking automated SLR tools that deliver higher quality reviews, configurable to the complexities of economic evaluations, and are scalable to handle the high volume of references. This business brief offers a SLR solution that is fit-for-purpose for the unique needs of health economics and outcomes research.
Why Are Cost Studies Gaining Momentum?
- Substantiate drug formulary inclusion for a private health care system
- Compare a new intervention against a threshold value for a country’s health system
- Determine efficient allocation of limited resources by a health technology assessment body
- Determine reimbursement level for a new drug by a payer
- Negotiate metrics in value-based pricing agreements
- Inform clinical care pathways to ensure optimized treatment, patterns of care, and resource allocation
Role of Systematic Reviews in Economic Models
In modeled CEA and BIM studies, the efficacy or effectiveness measures (e.g., outcomes) are derived from clinical trials, real world evidence, systematic reviews or meta-analysis. Systematic literature reviews are also used to synthesize the vast amount of cost-based studies, to enable clinical and policy-based decision-making.
Systematic literature reviews of economic analysis such as CEA and BIM must consider quality and bias risk to ensure valid output that is comprehensive, robust, transparent and reproducible.
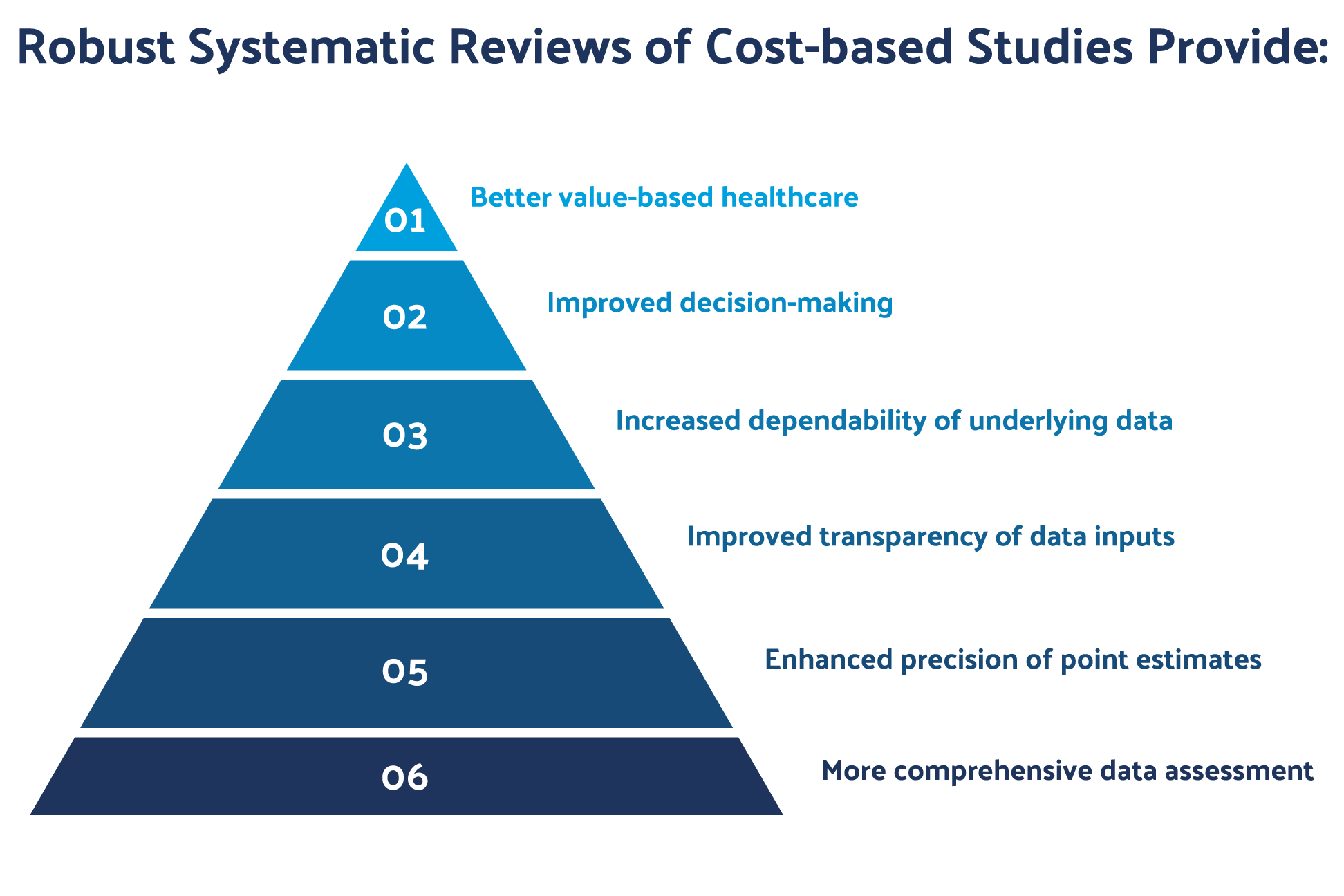
Figure 1: The diagram presents an example of how AI may be integrated into the review process.
In Figure 1, SLRs establish a standard evidence-based process that imbues greater levels of research rigor and transparency, all of which engenders a high degree of confidence in decision-making.
To achieve the highest quality SLR for cost-based studies, HEOR researchers may rely upon several approaches to inform their process:
- ISPOR Good Practices Report for Systematic Reviews with Costs and Cost-effectiveness Outcomes (Mandrik,2021)
- Checklists for quality and quality reporting of cost and cost-effectiveness studies (Wijnen, 2016)
- ISPOR CiCERO Checklist (Mandrik, 2021)
- Transparent reporting of methods and results by adherence to evidence-based reporting standards, such as PRISMA (Page 2021) for systematic review and CHEERS (Husereau, 2013) for health economic evaluation
- Automated tools that accelerate the delivery of high-quality literature reviews, such as DistillerSR
Cost Study Systematic Reviews and Critical Appraisal of Included Studies
HEOR researchers conducting reviews of cost studies should refer to the recently published Good Practices Report, “Critical Appraisal of Systematic Reviews with Costs and Cost-Effectiveness Outcomes,” which is based on a multidisciplinary task force convened by ISPOR (The Professional Society for Health Economics and Outcomes Research) (Mandrik, 2021). The report also includes the ISPOR Criteria for Cost-Effectiveness Review Outcomes (CiCERO) Checklist.
The guidance is intended to assist researchers, drug and health technology developers, and evidence users with guidance to interpret cost study quality and bias risk.
Value-based Decision-Making Powered by Systematic Literature Reviews
In addition to the above tools, HEOR researchers may use the Preferred Reporting Items for Systematic Reviews and Meta-Analyses (PRISMA), an evidence-based minimum set of items for reporting in systematic reviews and meta-analyses.
The PRISMA 2020 guidance was recently published (Page 2021). While PRISMA was originally developed for reviews evaluating randomized trials, the guidance is also used as a basis for reporting systematic reviews of other types of research, particularly evaluations of interventions.
In addition to PRISMA, HEOR researchers may utilize the Consolidated Health Economic Evaluation Reporting Standards (CHEERS) (Husereau, 2013), a reporting guideline to optimize the reporting of health economic evaluations, including CEA and BIM. A revision of CHEERS, called CHEERS II is under review and is expected to be released in early 2022.
For example, cost and cost-effectiveness studies may include several outcomes ranging from efficacy, effectiveness, safety, patient-reported outcomes, quality metrics, as well as resource utilization or costs. Two or more comparators may be included, and the assessments may span across multiple countries, therapeutic areas, variable time horizons, a range of scenarios or sub-populations, diseases and stages, as well as perspectives. Study selection and eligibility is complex, and grey literature may be included.
Study design can include model-based and/or empirical studies. Data volumes are typically sizable and data extraction is complicated. These realities often require multiple reviewers to accomplish the task, leading to valid concerns about errors and omissions. Organizations are mindful of the need for an audit trail for regulatory bodies and desire to publish the systematic review. Figure 2 provides a summary of key SLR challenges for economic evaluations. These challenges are addressed by the efficient, repeatable, scalable, and automated literature review process offered by DistillerSR.

Figure 2: Key challenges associated with SLRs for CEA and BIM.
The cloud-based platform also has a highly configurable and intelligent workflow, which allows HEOR professional to track all activity and coordinate work assignments.
For example, DistillerSR’s continuous and automatic AI re-rank uses machine learning to learn from the references you are including and excluding to automatically reorder the ones left to screen, putting the references most likely to be relevant in front of you. This means you find and include pertinent references much more quickly. AI classifiers can be trained to automatically answer questions, such as: “Is this a randomized controlled trial” or ”Is this an adverse event?” These classifiers can be applied to screening or assessment forms to answer targeted questions automatically. References are automatically imported to update a review using DistillerSR. Review tasks, meanwhile, are automatically assigned to the appropriate reviewer with intelligent workflows configured to the way your team works.
Automating elements of your CEA and BIM systematic literature reviews with DistillerSR leads to:
- Efficient identification of relevant evidence
- Continuously updated literature
- Faster time to completion
- Better team collaboration
- Fewer errors
Stakeholders, whether regulators, payers and health technology assessment bodies, clinicians, or journal reviewers, need to understand the systematic review protocol used to populate the model, if it is based on literature. Organizations that provide research for regulatory review must follow specific standards when it comes to reporting systematic literature review and economic models; audit trails are, therefore, essential for all systematic reviews.
With DistillerSR, everything is automatically tracked and documented. It is easy for an auditor or decision-making body to confirm that the literature review was conducted according to required standards and best practices. It allows stakeholders to trust the data and findings being presented.
An audit trail that utilizes user, date, and timestamps is as transparent as it gets. Also, the act of automatically recording everything makes sure everyone on the team is following the proper protocol. The major purpose of an audit trail, in this instance, is having the ability to answer questions efficiently and accurately in conformity assessments. For instance, ‘Who reviewed this reference?’ and ‘When was this work completed?’ are both questions that can be quickly answered thanks to an audit trail.
In addition to assisting with compliance requirements, an audit trail helps teams collaborate better and faster. Timestamping and user stamping ensures that every change made to the data is noted. That way, if there is an error, it can be found and corrected efficiently, with a full record of any corrections made.
In summary, audit trails help preserve data provenance, tracking all changes and preserving every version of the data throughout the process. These types of “digital forensics” are important for organizations that deal with data that informs policy and guidelines or organizations that could be the subject of legal proceedings.
Data extraction may be described as picking and pulling specific, relevant, and important information, or data, from literature. It is, essentially, the process of converting unstructured reference text to codified data that can be used in analysis. To ensure high-quality data, best practices dictate that two reviewers extract data in parallel and then compare results. This can be a highly labor-intensive and error-prone process.
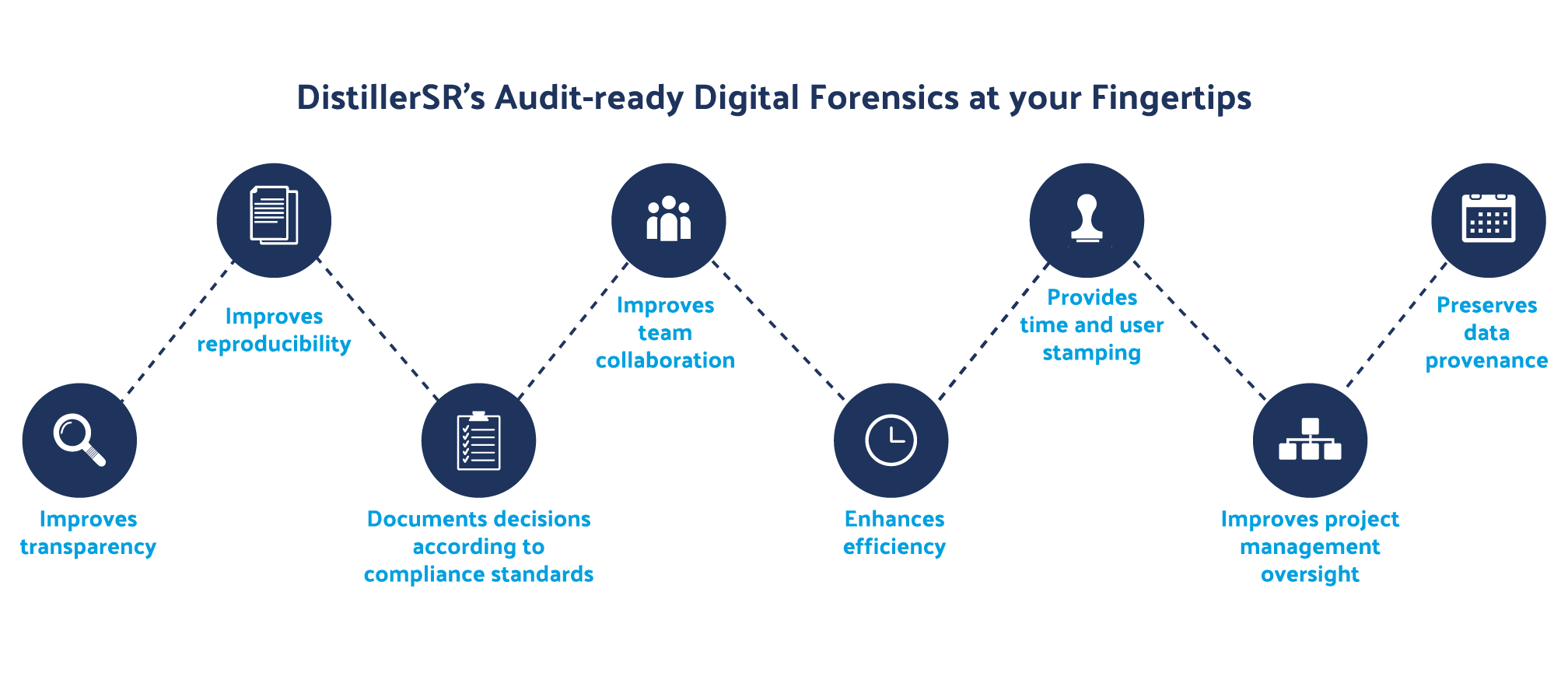
Figure 3: Auditable systematic reviews provide HEOR professionals with a higher degree of confidence in their data.
In most SLRs, groups commonly use one of three approaches: single reviewer, dual reviewers, or reviewer plus quality assurance (QA) in combination.
A single reviewer data extraction is efficient and less resourceintensive but does not have a second reviewer to catch errors or address initial review bias. This approach may be used for non-regulatory projects or scoping reviews.
A dual reviewer extraction process – the “gold standard” of SLR is commonly used for regulatory submissions, guideline development or other high-rigor projects. However, adding the second reviewer doubles the resource inputs. Dual reviews are challenging when they are complex. For example, this could include repeating data sets, such as capturing multiple time points for multiple outcomes within a single study. Data, for example, can differ between studies, or open-ended questions require consensus among reviewers.
The third approach, reviewer plus QA, is a hybrid comprised of data extraction initially done by a reviewer, followed by a second reviewer’s validation.
If discrepancies exist, the reference stays in the data extraction stage until resolved. This approach offers lower error and bias rates compared with the single reviewer approach and it is more efficient than the dual reviewer stage. False conflicts (slightly different information entered by two reviewers), and data syncing from complex repeating datasets are also less frequent.
However, proving true agreements is still challenging and can often involve bias, which may still exist from the first reviewer.
DistillerSR offers the overall benefit that all extracted and coded data for the entire review resides in one place, making validation and updates easier. Any changes to the data are version-controlled and extracted data can be exported using a variety of formatting and filtering options.
DistillerSR has built-in Automated Conflict Checking which enables a user to specify what constitutes a disagreement between data extractors and reviewers. This capability allows reviewers to identify and manage conflicts more accurately and efficiently. The approach is flexible enough to work with closed-ended questions, numeric fields, dates, and/or free-form text.
DistillerSR’s Quality Control (QC) function enables reviewers to have one person do the initial screening or data extraction, while a second person reviews their work for accuracy. If the QC reviewer does not agree with the initial reviewer, the reference will be flagged by DistillerSR as a conflict for resolution. Even when handling complex, repeating or hierarchical data, (such as follow-up data captured at multiple time points), DistillerSR easily identifies conflicts for efficient conflict resolution.
DistillerSR’s conflict management and quality control functionalities lead to:
- Faster, easier and configurable data extraction
- Better workflow
- Improved project management
- Greater efficiency
- Happier reviewers!

Figure 4: DistillerSR’s QC functionality facilitates validation of initial reviewers work by a secondary reviewer for a more time-efficient alternative to dual reviewing each citation.
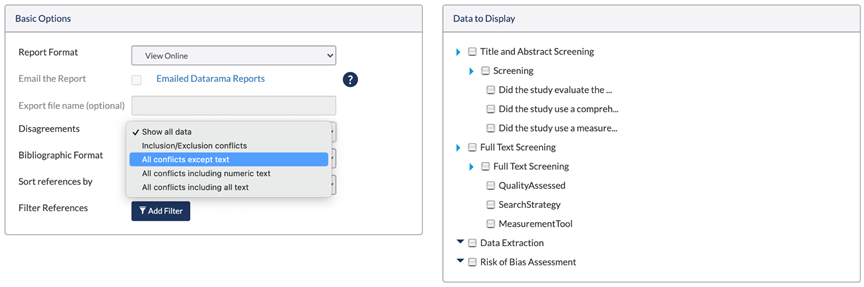
Figure 5: DistillerSR automatically identifies disagreements specified by your review criteria, making conflicts easy to address and manage.
- Khan ZM, Pizzo LT. Choosing the Right Path in HEOR Publishing. Value & Outcomes Spotlight. 2021(March/April):3. Accessed on May 13, 2021 from https://www.ispor.org/docs/default-source/publications/value-outcomes-spotlight/ispor_vos_april-2021_
onlinef38971b4b83541df9f82ba8532825509.pdf?sfvrsn=f1bc3062_0 - Mandrik O, Severens JL, Bardach A, et al. Critical Appraisal of Systematic Reviews With Costs and Cost-Effectiveness Outcomes (ISPOR Report). Value in Health. 2021 April 1;24(4):463-472. DOI: https://doi.org/10.1016/j.jval.2021.01.002
- Wijnen B, Van Mastrigt G, Redekop WK, et al. How to prepare a systematic review of economic evaluations for informing evidence-based healthcare decisions: data extraction, risk of bias, and transferability (part 3/3).Expert Rev Pharmacoecon Outcomes Res. 2016; 16: 723-732 https://pubmed.ncbi.nlm.nih.gov/27762640/
- Page MJ, McKenzie JE, Bossuyt PM, et al. The PRISMA 2020 statement: an updated guideline for reporting systematic reviews. BMJ 2021; 372 doi:https://doi.org/10.1136/bmj.n71 (Published 29 March 2021)
- Husereau D, Drummond M, Petrou S, et al. Consolidated health economic evaluation reporting standards (CHEERS)—explanation and elaboration: a report of the ISPOR Health Economic Evaluations Publication Guidelines Good Reporting Practices Task Force. Value Health. 2013;16(2):231-250. https://pubmed.ncbi.nlm.nih.gov/23538175/
Download the PDF version of this business brief.
Related Resources
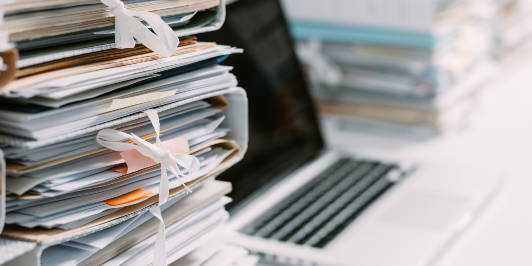
Case Study
With as many as 40,000 references per project, Medlior, a HEOR consultancy, used DistillerSR to more accurately and efficiently manage their literature reviews.
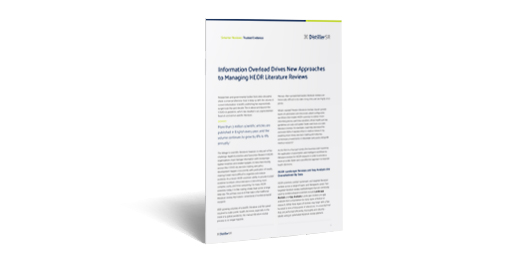
Business Brief
Information Overload Drives New Approaches to Managing HEOR Literature Reviews.

Webinar
How Information Overload is Driving New Approaches for HEOR Systematic Literature Reviews.