DistillerSR AI
Scientifically Validated AI
DistillerSR is a pioneer in the use of AI for literature reviews.
Since 2016, customers have trusted DistillerSR AI to accelerate
the completion and enhance the quality of their reviews.
Purpose-built GenAI for Literature Reviews
DistillerSR Smart Evidence Extraction (SEE) automatically finds, suggests, extracts and links data from scientific journals to answer closed and open-ended questions, including numerical content, sentiment analysis, risk of bias information, and study summaries.
Accurate and Reproducible
Auditable
Human-in-
the-Loop
Intuitively built into DistillerSR’s workflow to ensure users have oversight into the suggested answers and links to the data source.
Copyright and Privacy Compliant
AI That Learns From Your Data
DistillerSR AI autonomously trains itself on your data as you work. After only a few references, it quickly learns to recognize what references/studies/articles are relevant and then begins assisting with the screening process, reducing time to literature review completion by as much as 70%.
The diagonal line represents traditional screening methods while the green line represents the time saved with continuous AI reprioritization.
Faster Evidence Generation
Literature
Prioritization
Re-rank learns from your reviewers and reprioritizes references based on how you include and exclude them.
Automated
Quality Control
Validate reference inclusion and exclusion decisions made by humans or AI. Find potential errors in seconds.
More Efficient
Reviews
Accessible Domain Specific AI
DistillerSR AI lets you easily build and deploy custom AI Classifiers using data you are already collecting. AI Classifiers can then be used to automatically label references, identify key elements in a paper, or serve as a second reviewer. They produce predictable and consistent outcomes, explainability and, if required, streamlined human-in-the loop validation.
Accelerated
Pre-Screening
Start evaluating and extracting data from citations sooner by automatically pushing references to later stages of the review.
Workload
Triage
Automate workload assignment management to fully leverage your subject matter experts and assign references to particular forms or reviewers sooner.
AI Quality
Control
Increase the thoroughness of your reviews by having classifiers operate in tandem with your reviewers to double-check how you’ve categorized citations.
Eli Lilly’s Assessment of DistillerSR AI
At the professional society for health economics and outcomes research (ISPOR) Europe 2023 Conference, Eli Lilly Services India concluded that applying DistillerSR AI in title-abstract screening reduced 67% of human efforts (hours) irrespective of the type of literature review. It also reported that DistillerSR AI had a mean accuracy score of 90% for traditional systematic and targeted literature reviews.
DistillerSR AI and LLMs: What the Future Holds
Large language models (LLMs) are poised to play a critical role in literature reviews. Recognizing this, DistillerSR is heading an international consortium of companies, government agencies and academic institutions with the goal of fully automating the extraction of text and data from scientific literature. This undertaking is combining the scaling power of deterministic AI, large language models (LLM), and other technologies to produce reliable, explainable and fully automated results. This project is being funded by a number of national governments as well as the private sector, DistillerSR, Philips, University of Leiden, Synerscope, Ossur, Adelphi Group, Biotronik, and the Fraunhofer Institute for Experimental Software Engineering have joined forces in this initiative.
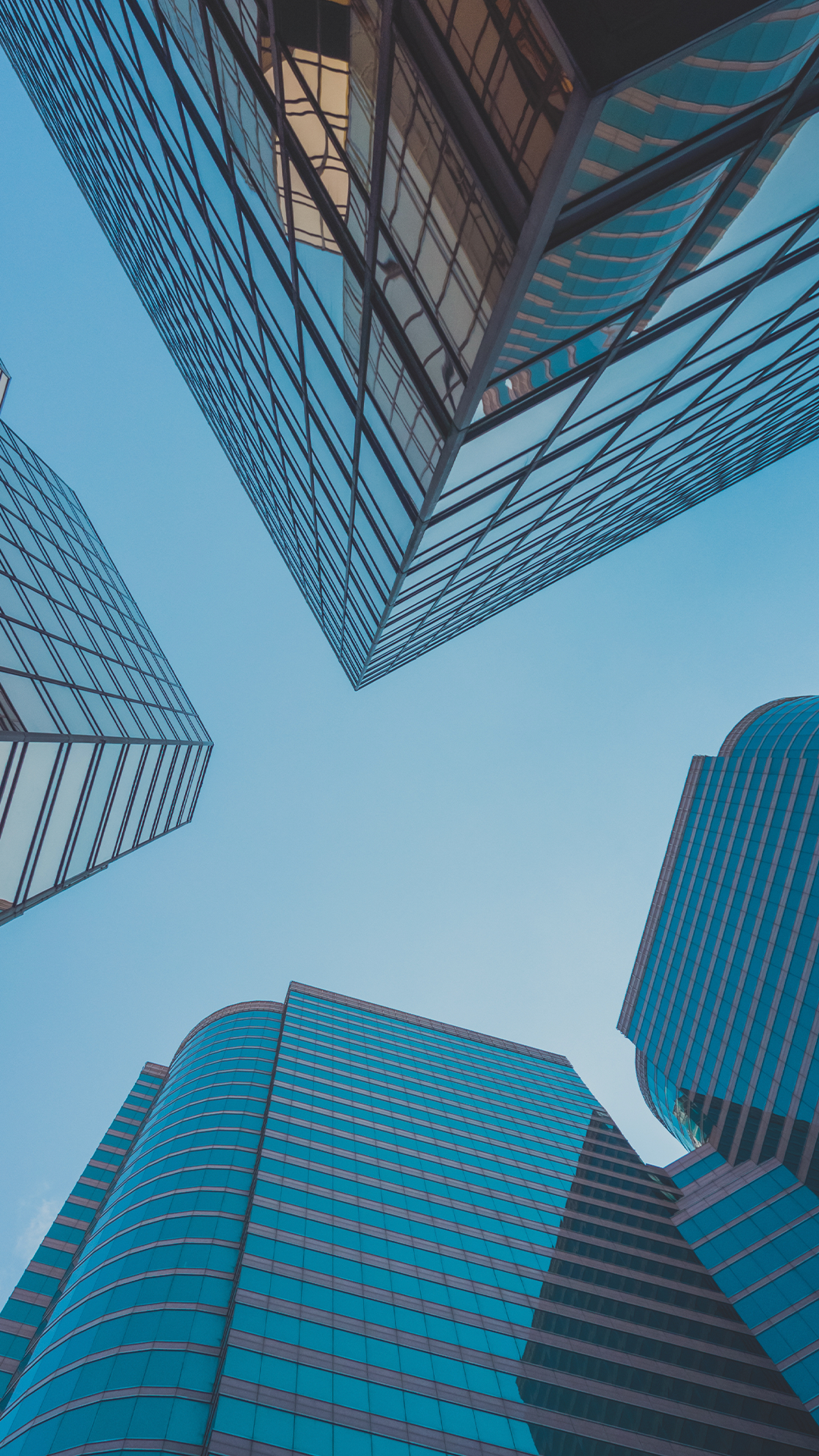
With industry, regulatory and academic stakeholders working together, consortium members believe these technologies, trained, and validated to therapeutic and healthcare domains, will allow academic and commercial research to identify risk of bias, establish contextual longitudinal assessments of data residing in non-scientific or technical literature, and identify and characterize commonalities and causal relationships across data sources – delivering clinical evidence faster to physicians, regulatory bodies and health authorities.
Scientifically Validated AI For Literature Reviews
Stefano Cagnonia, Vieri Emilianib, Gianfranco Lombardoa, Wynand Alkemac, Carlijn Hooijmansd 2023
Error rates of human reviewers during abstract screening in systematic reviews
Zhen WangID, Tarek Nayfeh, Jennifer Tetzlaff, Peter O’Blenis, Mohammad Hassan Murad 2020
Guidance for using artificial intelligence for title and abstract screening while conducting knowledge syntheses
C Hamel, M Hersi, SE Kelly, AC Tricco, S Straus, G Wells, B Pham, B Hutton 2021
An evaluation of DistillerSR’s machine learning-based prioritization tool for title/abstract screening – impact on reviewer-relevant outcomes
C Hamel, SE Kelly, K Thavorn, DB Rice, GA Wells, B Hutton 2020
Evaluating the efficacy of artificial intelligence tools for the automation of systematic reviews in cancer research: A systematic review
X Yao, MV Kumar, E Su, A Flores Miranda, A Saha, J Sussman 2023
Application of Artificial Intelligence in Literature Reviews
Shiva Kumar Venkata, Sravani Velicheti, Vinayak Jamdade, Sreeja R, Monika Achra, Kushal Kumar Banerjee, Chandreyee Dutta Gupta, Michael Happich, Annabel Barrett Eli Lilly Services India Private Limited India, Eli Lilly and Company United Kingdom 2023
Using an artificial intelligence tool can be as accurate as human assessors in level one screening for a systematic review
JK Burns, C Etherington, O Cheng-Boivin, S Boet 2021
Related Resources
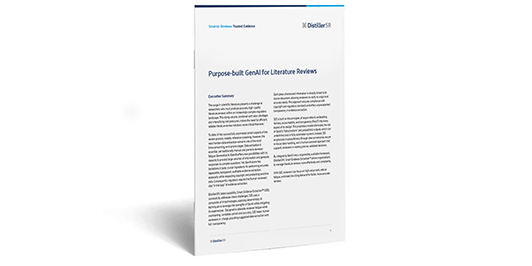
Purpose-built GenAI for Literature Reviews
Learn how reviewers can focus on high-value work, reduce fatigue, and meet the rising demand for faster, more accurate reviews.

Health Agency
Learn how this Health Agency used AI to reduce COVID-19 citation screening and data extraction by 50%.