Solution Brief
The Case for Artificial Intelligence in Systematic ReviewsEvidence syntheses, essential to informing decision-making, are resource intensive and time consuming. Systematic reviews (SRs) take an average of 67.3 weeks to complete and publish and require considerable resources to identify relevant evidence.1 Published scientific literature has increased by 8-9% each year,2 with over 3 million scientific articles published annually in English alone.3 As the yield from literature searches grows, the screening burden increases, requiring more time, additional funding, and larger review teams. These factors introduce more opportunities for human error, which has been shown to be substantial.4
Faster and more accurate SRs could help decrease research waste, estimated to be up to 85%,5,6 by enabling more timely decision making and reducing unnecessary investments in redundant and poorly designed medical research. Artificial intelligence (AI) can play a critical role in automating, accelerating, and reducing the costs associated with knowledge syntheses.
A key application of AI is the use of active machine learning (AML), which can help identify relevant records over 50% sooner, on average, than traditional methods.
As a result, AI can hasten researchers’ efforts to inform time-sensitive health policies, clinical practice guidelines, and regulatory submissions.
Growing AI Maturity for SRs
AI is being explored for many aspects of the knowledge synthesis process. The current focus of AI is on screening using AML. These systems require quality input from humans (i.e., “human-in-the-loop”). AML can empower research teams to identify relevant evidence more efficiently. Doing so allows the subsequent SR steps to be completed sooner or concurrently and can reduce screening burden. AI can also modernize the process of title/abstract screening, identify human error, and facilitate more timely delivery of evidence to end users.

Shifting SR Traditional Processes: A Use Case
Typically, SRs and other literature surveillance tasks are performed using a rigid sequential process, whereby reviewers first screen all records identified from the search based on the information provided in the bibliographic record (e.g., title and abstract). Once complete, full texts for any records deemed potentially relevant are then procured and are further reviewed.
Continuous AI reprioritization, an application using AML, starts automatically when a new project is created and can be disabled/enabled as needed. It can offer important benefits for research teams conducting knowledge syntheses.
(1) More efficient use of methodological expertise: When relevant records are identified sooner, teams can begin subsequent review steps (e.g., full-text procurement and screening, data extraction and analysis) earlier. This enables methodological and content experts to contribute more substantially earlier to tasks requiring additional skills while screening continues for records which are less likely to be relevant.
(2) Reduce screening workload: Once a threshold has been met (e.g., a predicted 95% of the relevant records have been identified), records not likely to be relevant may be left unscreened, or a different screening approach (e.g., single-reviewer screening) may be used.
(3) Expedite review updates, living reviews and literature surveillance: SR professionals can leverage the advantages of a system primed with previous screening decisions.
To maximize performance, teams should consider strategies to build and maintain accurate training sets, to ensure that the AML learns from the data and produces accurate results.
DistillerSR includes multiple AI functions to support the review process, three of which are described below. They may be used independently; however, the greatest gains in efficiency are achieved when used together:
(1) Continuous AI reprioritization: Re-orders study records such that they are presented by order of predicted relevance to the review. This process is performed in the background, and AML occurs while screening is conducted. Include/exclude decisions build an iteration (a set of records) which informs the overall training set (i.e., the compilation of all iterations). After each iteration, new screening decisions further inform the re-ordering of unscreened records and the system learns as
screening progresses.
(2) Predictive reporting tool:
Provides a conservative prediction of the number of relevant records not yet identified and increases in accuracy as screening progresses. It also presents a graphical presentation of screening progress; the inclusion likelihood scores of the remaining unscreened records; and the details of each
iteration. This empowers the team to maintain a ‘pulse’ on the project status and shift tasks where needed.

(3) Error checking: Detect and correct screening errors. AI audit and AI simulation help identify potentially incorrectly excluded and incorrectly included records. Both offer methods to easily correct screening errors if identified. When used regularly, these tools can also promote cleaner training sets and can further increase the performance of continuous AI reprioritization.
AI’s Impact on SRs: A Balanced Approach
The AML algorithm in DistillerSR has been optimized and validated with hundreds of reviews to date and has shown substantial efficiencies to identify relevant records.

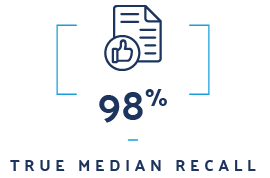
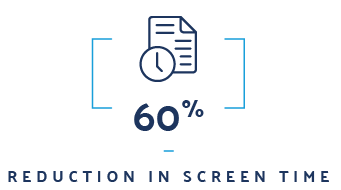
The AML algorithm in DistillerSR has been optimized and validated with hundreds of reviews to date and has shown substantial efficiencies to identify relevant records. Below is an example of a customer’s application of continuous AI reprioritization vs. conventional screening.

Summary
AI can optimize SRs and SR updates by creating efficiencies across the review process, minimize screening errors, and potentially can reduce the screening burden where modified screening approaches are appropriate. DistillerSR’s AI functionality is designed to increase the effi ciency of knowledge syntheses and provide teams with transparency on the performance of AI for their reviews. These applications have been tested across a range of reviews, can be used with little risk and most teams will see measurable benefits.
AI’s application also creates opportunities for other considerations, such as using broader literature search approaches, where less concessions may be needed to reduce the search yield to meet budget or timeline restrictions. AI provides SR professionals with an additional, powerful capability to inform scientific and medical research faster and more cost-effectively. This can have a profound impact on the speed with which healthcare and research decisions can be made.
- Borah R, Brown A, Capers P, Kaiser K. Analysis of the time and workers needed to conduct systematic reviews of medical interventions using data from the PROSPERO registry. BMJ Open. 2017;7:e012545.
- Landhuis E. Scientific literature: Information overload. Nature.
2016;535(7612):457-458. doi:10.1038/nj7612-457a - Johnson R, Watkinson A, Mabe M. The STM Report: An overview of scientific and scholarly publishing (Fifth Edition). The Hague: International Association of Scientific, Technical and Medical Publishers; 2018. Available at: https://www.stmassoc.org/2018_10_04_STM_Report_2018.pdf. Accessed October 8, 2020.
- Wang Z, Nayfeh T, Tetzlaff J, O’Blenis P, Murad MH. Error rates of human reviewers during abstract screening in systematic reviews. PLoS ONE.
2020;15(1):e0227742. doi:10.1371/journal.pone.0227742 - Chalmers I, Glasziou P. Avoidable waste in the production and reporting of research evidence. Lancet. 2009;374(9683):86-89. doi:10.1016/S0140-6736(09)60329-9
- Glasziou P, Chalmers I. Research waste is still a scandal—an essay by Paul Glasziou and Iain Chalmers. BMJ. 2018;363. doi:10.1136/bmj.k4645