Business Brief
Information Overload Drives New Approaches to Managing HEOR Literature ReviewsResearchers and governmental bodies from every discipline share a universal dilemma: How to keep up with the volume of current information. Scientific publishing has exponentially surged over the past decade. This is above and beyond the COVID-19 pandemic, which has resulted in an unprecedented flood of coronavirus-specific literature.
With growing volumes of scientific literature and the speed required to make public health decisions, especially in the midst of a global pandemic, the manual literature review process is no longer feasible.
What’s needed? Modern literature reviews require greater levels of automation and structured, albeit configurable, workflows that enable HEOR scientists to deliver more informed, precise, and time-sensitive critical health policies guidelines at scale and speed. Faster and more accurate literature reviews, for example, could help decrease the estimated 85% of wasted effort in medical research, by enabling more timely decision making and reducing unnecessary investments in redundant and poorly designed medical research.2
As the first in a four-part series, this business brief examines the application of automation and intelligent workflows to literature reviews for HEOR research in order to provide a more accurate, faster and cost-effective approach to improve health decisions.
HEOR Landscape Reviews and Gap Analysis Are Overwhelmed By Data
HEOR scientists conduct systematic and targeted literature reviews across a range of topics and therapeutic areas. Two targeted literature review methodologies that are commonly used by evidence-based scientists include landscape reviews and gap analyses. Landscape reviews and gap analyses form a foundation for many types of follow-on research. While these types of reviews may begin with a few hundred to tens of thousands of references, it is essential that they are performed efficiently, thoroughly and robustly – ideally using an automated literature review platform.
Landscape reviews are frequently used in HEOR to understand clinical, economic, and patient-reported outcomes for designing future clinical or real-world evidence studies. These reviews may clarify where better interventions are needed in a particular therapeutic area. These targeted reviews may also be used in health technology assessment (HTA) or payer-facing dossiers, or for identifying cost-effectiveness models. Landscape reviews integrate evidence from the literature to inform the value demonstration plan to maximize market access success with providers, payers, regulators, and patients.The goal is rapid categorizing and characterizing of information, all of which lend themselves to a structured, contained workflow of a literature review platform.
Gap analysis, meanwhile, provides a holistic collation of currently available evidence and identifies data gaps. It is similar to a needs assessment, but allows for a more standardized process to determine the gap in knowledge based on the quality of available evidence. Gap analysis is also used to develop a research plan that demonstrates the value of an intervention and, hopefully, attains market access. By defining “where are we today?”, evidence gaps provide a roadmap for data needs. Evidence strength and quality, portrayed as a heat map, facilitate interpretation and usability of gap analyses.

The traditional conduct of literature reviews, nonetheless, is under considerable pressure on two fronts. First, growing volumes of scientific literature and the increasing demand to conduct more systematic reviews requires managing more references and larger teams, often remotely, using processes that make it difficult to produce work quickly, accurately, and cost-effectively. In many cases, manual literature reviews require extensive administration to send emails, edit spreadsheets, attach documents, and wait for replies – all potentially error-prone approaches. Numerous reviews and the volume of incoming references requires more staff and time spent on the manual administrative coordination of reviews. Secondly, manual literature review processes do not meet the modern standard for transparency and reproducibility underpinned by proven methodologies. The sheer volume of data and logistics involved in a manual review makes catching errors, comparing results, and auditing findings a huge challenge.
These solutions provide a basic set of capabilities that are neither optimized to support different review types, flexible protocol changes, or large reference sets.
- Identify outcomes that can be used in future research studies
- Guide strategy and evidence-basis for value propositions
- Provide background for HTA or payer dossiers
- Identify inputs for disease or cost-effectiveness models
- Facilitate value demonstration planning for market access
Gap Analysis Lit Reviews
- Defines data gaps to inform a research plan
- Characterizes evidence gaps by type of outcome, study design, customer, or geography, among others
- Used to develop strategic plan for value demonstration and market access
- Need summary to lit review re applicability to process
Literature reviews, like landscape or gap analyses, may need to rely upon numerous data providers and sources of research. As such, it’s not uncommon that initial search strategies return 5,000 references from hundreds of thousands of publications. Better, more thorough reviews result in improved decision-making, superior follow-on research plans, and accelerated market access.
Using DistillerSR, reviewers can configure auto-alerts with their preferred data providers, such as PubMed, Ovid or EMBASE, to automatically import literature into the software platform —allowing for the widest search of literature while reducing the time associated with conducting it. This continuous process allows living reviews to be updated with new references as they become available and automatically assigns them to the right reviewers. Once imported, the references can be de-duplicated and automatically assigned to appropriate reviewers. Reviewers then get notifications when new references require screening. DistillerSR can readily scale for literature reviews of all sizes, supporting more than 675,000 references per project.
Freely available full-text documents can also be automatically retrieved and added to reviews, and teams can leverage subscriptions to Article Galaxy and RightFind to order references directly from within DistillerSR. For organizations using e-libraries and DOI.org, reviewers can access source materials and full text documents, wherever they are stored, directly while reviewing.
Manual screening of these references may be too time-consuming, particularly with mission critical projects. To address this, DistillerSR seamlessly integrates AI into reference screening, error checking, and classification workflows in two complimentary ways.
First, DistillerSR’s AI monitors the screening process and learns to recognize the attributes of references that reviewers include in their projects. DistillerSR then uses this knowledge to continuously bring the most relevant references to the top of reviewers’ lists automatically and allows review teams to screen the most relevant references first. This has a dramatic impact on reducing the overall screening burden. Though time savings will vary by project, DistillerSR has identified 95% of relevant records on average 60% sooner using AI, enabling researchers to start work on later stages of the review (e.g. full-text screening, data appraisal and extraction) sooner.
Second, DistillerSR includes a predictive reporting tool that informs researchers about the likelihood of relevance of the remaining unscreened references.
This enables review teams to better allocate review resources and to consider stopping rules for screening. DistillerSR also allows a reviewer to create and reuse AI classifiers to identify specific reference attributes. For example, AI classifiers could be used to identify a specific intervention, PICO element or study design of a reference, further automating the review process.
For one federal government agency during the COVID-19 pandemic, reviewers monitoring newly published scientific literature would see as many as 1,400 new references per day. According to the agency’s internal analysis, manually screening and extracting data from references took a human reviewer up to 10 minutes per citation to complete, but with the help of DistillerSR’s AI that time was cut to 3-5 minutes. In total, AI enabled reviewers to reduce the time needed to complete their work by 50% to 78% each day.
With DistillerSR relevant references are found sooner and can quickly move forward in the review process. AI-assisted screening and error checking, meanwhile, significantly reduces overall screening times.
“DistillerSR not only makes the screening process simple, but also allows for creative and flexible solutions when managing massive volumes of evidence and broad inclusion criteria.”
Tracking all review activity and making it easy to trace the provenance of every cell of data, delivers total transparency and auditability into the review process. With reviewers potentially working across the globe, it’s critical for HEOR teams to stay on the same page. While traditional “spreadsheet” methods for literature reviews are limited in terms of facilitating collaboration, error detection, and version control, DistillerSR enables team members to work on the same project simultaneously without the risk of duplicating work or overwriting each other’s results.
For example, some reviews may not apply dual screening to their process. The benefit of an embedded audit trail ensures all versions are tracked, so teams know what was done and changed by whom and when. Team leaders can then monitor the progress of the review and resolve reviewer conflicts in real-time. Configurable workflow filters ensure that the right references are automatically assigned to the right reviewers, and DistillerSR’s cross-project dashboard allows reviewers to monitor to-do lists for all projects from one place.
In addition, it is common for HEOR-based literature reviews to require protocol changes in mid-stream. Very often, this results in re-reviewing, re-searching, and re-checking references. DistillerSR allows protocol changes to be made whenever they are needed and it allows those changes to be retroactively applied to references that have already been processed, thus making it easy for researchers to pivot and adapt as circumstances change or more information emerges.
The growing body of research and the pressing need for quick evidence reviews to drive decision-making and policy has changed the way we conduct literature reviews. Research scientists, such as those focused on health outcomes and resource utilization, need solutions that improve the quality of their literature reviews, while increasing efficiency and resource management.
A recent article in BMC’s Medical Research Methodology reported DistillerSR reduced article screening burdens by as much as five person-weeks on a single project. This illustrates the time savings to be gained by incorporating automation into systematic reviews, allowing researchers to manage growing volumes of new data and time pressures required to rapidly assess new medical treatments.
- Johnson R, Watkinson A, Mabe M. The STM Report: An overview of scientific and scholarly publishing (Fifth Edition). The Hague: International Association of Scientific, Technical and Medical Publishers; 2018. Available at: https://www.stm-assoc.org/2018_10_04_STM_Report_2018.pdf
- Glasziou, P., Altman, D. G., Bossuyt, P., Boutron, I., Clarke, M., Julious, S., … & Wager, E. (2014). Reducing waste from incomplete or unusable reports of biomedical research. The Lancet
- C. Hamel, S. E. Kelly, K. Thavorn, D. B. Rice, G. A. Wells & B. Hutton (2020) An evaluation of DistillerSR’s machine learning-based prioritization tool for title/abstract screening—impact on reviewer-relevant outcomes. BMC Medical Research Methodology.
Download the PDF version of this business brief.
Related Resources
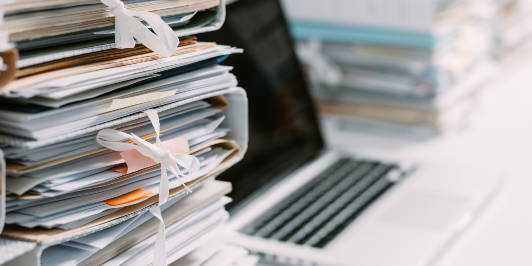
Case Study
With as many as 40,000 references per project, Medlior, a HEOR consultancy, used DistillerSR to more accurately and efficiently manage their literature reviews.

Webinar
How Information Overload is Driving New Approaches for HEOR Systematic Literature Reviews.
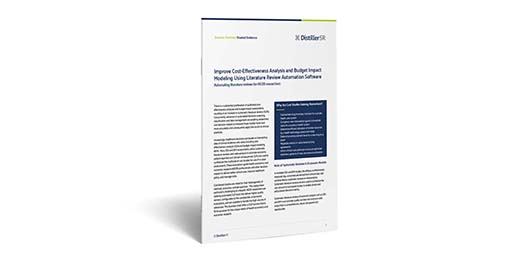
Business Brief
Improve Cost-Effectiveness Analysis and Budget Impact Modeling Using Literature Review Automation Software