Business Brief
Enterprise Evidence Management for Trusted Healthcare Decision MakingExecutive Summary
A centralized evidence management strategy that breaks down data silos and fosters cross-functional collaboration can significantly enhance product development and decision-making. This strategy includes establishing a centralized evidence repository and standardizing data collection and analysis processes across functions, which not only reduces redundancy and costs but also ensures rapid access to crucial data, supports regulatory compliance, and facilitates effective decision-making.
The implementation of such a strategy relies heavily on the right technology ecosystem. Platforms that enable real-time collaboration and centralized evidence storage and retrieval will allow secure knowledge sharing across various organizational levels and external partners. By moving towards a unified evidence management framework, pharmaceutical and medical device companies can meet the rising demands for data transparency, effectively utilize RWE, and drive growth, compliance, and improved outcomes.
Download the PDF of this Brief
Introduction
Patients, healthcare providers, payers, and regulatory bodies are increasingly demanding that the healthcare manufacturers provide robust clinical data to prove their products’ clinical safety, performance, and economic value. Therefore, generating, analyzing, and distributing data in an effective manner is critical to pharmaceutical and medical device companies. This can be a costly endeavor, with clinical trials costing millions of dollars and taking years to complete. Deloitte estimates the cost to develop new drugs, from research and discovery through post-market, at approximately $2.3 billion, and the average time to complete the process at 15 to 20 years.1 For medical devices, that cost can be $25 to $200 million, depending on the complexity and invasiveness of the device.2 Moreover, it can be impractical to run a clinical trial for every aspect of a product. As a result, effective data management and real-world evidence are increasingly critical to the healthcare industry as companies look to provide the critical evidence they need to support their products.
Real-world evidence includes surveys, patient electronic health data or feedback, registries, and published clinical and scientific literature that does not depend on sponsored clinical trials.3 The diverse nature of this information means it can be useful to and collected by multiple functional teams in an organization. Cross-functional review can thus benefit the whole organization, but only when that data is rapidly and effectively shared. Unfortunately, barriers among functions often create issues for data sharing.
Siloed organizations are created when expertise is aggregated into functions or departments. It can inhibit product development when bridges are not built between functional areas, especially around data-sharing. According to one study, teams specializing in data and analytics that built effective data sharing systems were 1.7 times more effective at their role and at presenting demonstrative, verifiable data to leadership.4 Though the importance of cross-functional collaboration has long been recognized, lack of data transparency continues to be a significant issue for many organizations. Developing effective strategies for generating and sharing data across an organization is thus crucial to ensuring product success and better patient outcomes.
Siloed Evidence Collection: A Barrier to Trusted Research
Functions across organizations collect scientific and clinical data for a variety of reasons, from research and development to regulatory needs to reimbursement, market access, and product positioning. Very often, the same literature is being purchased repeatedly across the business for several different activities, but the output is not being socialized or shared.
Additionally, teams or departments are storing the data in different systems like SharePoint, spreadsheets or various databases, private file systems, or outside software solutions. These information silos can also be a tremendous cost to a business. In 2016, IBM conducted a study on the cost of bad data and found that it represented a $3.1 trillion annual loss to the US economy.5 A survey by research firm Gartner confirmed the high cost at the organization level, finding that “organizations believe poor data quality to be responsible for an average of $15 million per year in losses.”6
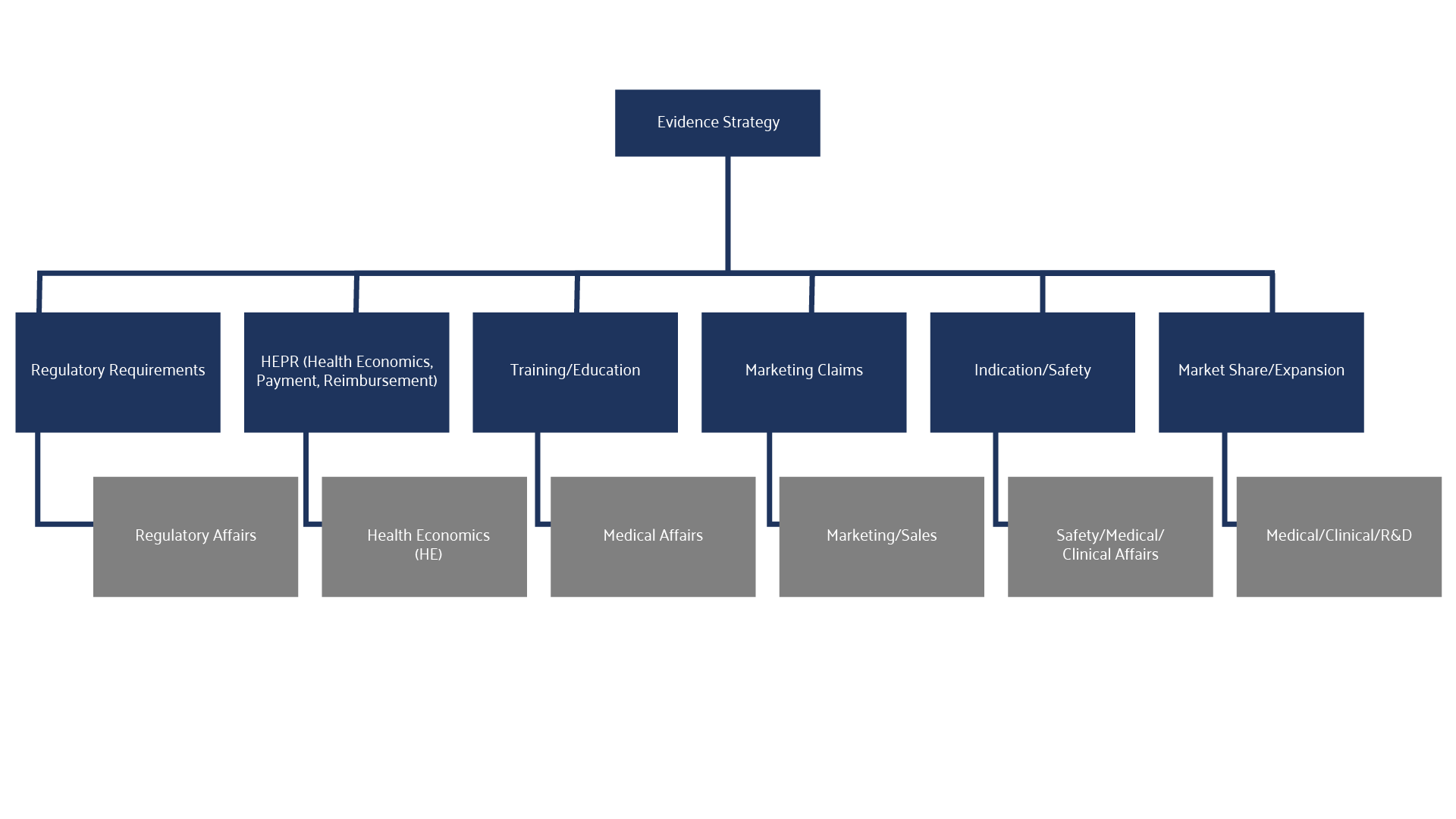
Examples of ineffective data-sharing and avoidable rework are not difficult to come by. Regulatory affairs professionals in medical device companies create large regulatory dossiers that detail and synthesize the clinical data on the safety and performance of their product as well as on competitor devices — an enormous task that can take up to 500 hours to complete. At the same time, the company’s marketing department may be reviewing competitor data for competitive messaging or new business opportunities, and healthcare economic groups may want to demonstrate that their device meets state-of-the-art and clinical guidelines to qualify for reimbursement. Since every function is working independently and not sharing data, these teams will unknowingly review the same literature and spend an additional 30 to 50 hours to complete the work. Meanwhile, clinical and medical affairs teams must provide compelling and unbiased evidence to their healthcare partners and key opinion leaders by creating summary documents and presentations on therapeutics, medical conditions, and best practices, with each review taking another 10 to 20 hours. All of these groups are accessing and interpreting the same data, but often without sharing the information they glean from it.
The reasons for these functional silos are varied. Different functions may not know what the other is doing, or they may be looking to “control” the data. Groups or functions may also become competitive with one another, and look to keep critical information to themselves as a way to secure their business needs or budget. There are legal and regulatory reasons too, for why data may not be shared between different groups, and not knowing what can be shared with whom often leads to not sharing any information at all. The consequences range from missed opportunities, regulatory findings due to inconsistent documentation or lack of evidence, and extra time and resource costs associated with multiple people performing the same task or reviewing the same data.
Establishing an Enterprise Evidence Strategy
Healthcare organizations must find ways to break down barriers around isolated information and evidence and view sharing data as an opportunity for growth instead of a risk. Since silos are a natural result of scale and business complexity, teams must be deliberate in their efforts to foster cross-collaboration, including standardizing literature reviews and data gathering both internally and externally with potential partners.
An enterprise evidence strategy sets the stage for information-sharing by enabling all key players to proactively establish what the evidence for a project or product will be.
The approach involves the creation of a cross-functional team with leadership governance that plans, executes, and reevaluates evidence needs across the business throughout the product lifecycle. This creates a cohesive structure for a body of trusted evidence that can be accessed across the organization and, if need be, shared with external partners. Common standards/ontologies meanwhile, support a governance model for managing evidence throughout an organization.
The advantages of establishing a cross-functional approach become apparent soon after implementation. Along with the most obvious benefit of reducing resourcing and costs associated with data rework, having a global evidence strategy helps ensure consistency across documentation and functions. This is critical to regulatory compliance while ensuring rapid and accurate access to information by key decision-makers and medical communications teams. It also helps to quickly identify evidence gaps that need to be filled by clinical trial or real-world evidence collection.
A recent article by McKinsey and Co. cites an organization that “identified no fewer than 18 critical evidence gaps that were not previously spotted by individual functions”7 for an oncology therapy. Establishing an evidence strategy generation enabled the company to more effectively budget for urgent and long-term needs, reduce the need to resource investigator-initiated research, and identify synergies between health economics and outcomes and clinical affairs in post hoc trial analysis. In a follow-up survey, over 80% of the people involved in the evidence strategy development reported “increased synergies in cross functional evidence generation” as a result.
Systematic Collection, Curation, and Reuse of Literature-Based Evidence
Any organization undertaking a unified evidence strategy needs a centralized evidence repository. As mentioned earlier, most siloed functional teams tend to organize and store their data separate from one another, with little to no cross-sharing of their individual databases. Furthermore, there is often little consistency between those databases, making data aggregation and reuse extremely difficult. A key part of a successful global evidence strategy is, therefore, an effective platform to share data and to house a centralized evidence library or repository of the available published literature and evidence that can be reused consistently across functions. The platform should also establish a standardized approach to data collection, analysis, and interpretation. When functions are siloed, clinical or medical safety teams may collect, treat, or interpret data differently than regulatory or post-market surveillance teams, and marketing may have a different take than leadership on what the evidence suggests, leading to misalignment of approved regulatory market messaging. A global evidence strategy combined with a standardized, centralized library of published literature and analysis can reduce, if not altogether eliminate, those barriers. Decisions are made with speed and efficiency because the right person has access to the right data at the right time to effect change, drive decisions, and have impactful conversations with customers. Healthcare companies need to be both active and proactive in sharing data and developing systems for evidence management. This requires them to champion cross-functional evidence strategies at the leadership level, establish teams to govern these new processes and to manage the change, and invest in data platforms that will support evidence curation, analysis, and sharing.
What Is Enterprise Evidence Management?
Enterprise evidence management is the centralized collection and curation of literature review data that can be mined and shared among stakeholders within an organization to improve consistency and collaboration, ultimately, reducing operational risks and costs.
This framework creates a competitive advantage for companies that understand how to standardize data collection and analyses to best capture the value of literature reviews. Standardizing processes leads to consistent results, even when multiple users may perform the same literature reviews, which means the data feeding into regulatory submissions will also be consistent and trusted.
Companies use centralized evidence management to share data across business functions and create a consistent approach for data collection and analysis, from risk analysis to post-market surveillance, throughout the device/drug development lifecycle. Regardless of size, all organizations can benefit from the ability to rapidly review and justify their product portfolio by leveraging high-quality data through evidence reuse.
The Way Forward: Trusted Enterprise Evidence
Our analysis of DistillerSR customers identified key stages of evidence management. The Enterprise Evidence Management Maturity Model illustrated in figure 2 provides a best practices framework for organizations to build and improve the centralized management of evidence extracted from literature reviews.
In support of efficient, timely, and accurate evidence-based decision-making, this model maps AI-enabled automation and evidence management capabilities versus their impact on value creation for the business. It also helps organizations benchmark their capacity to connect business outcomes, such as productivity and profit margins, to the generation of evidence.
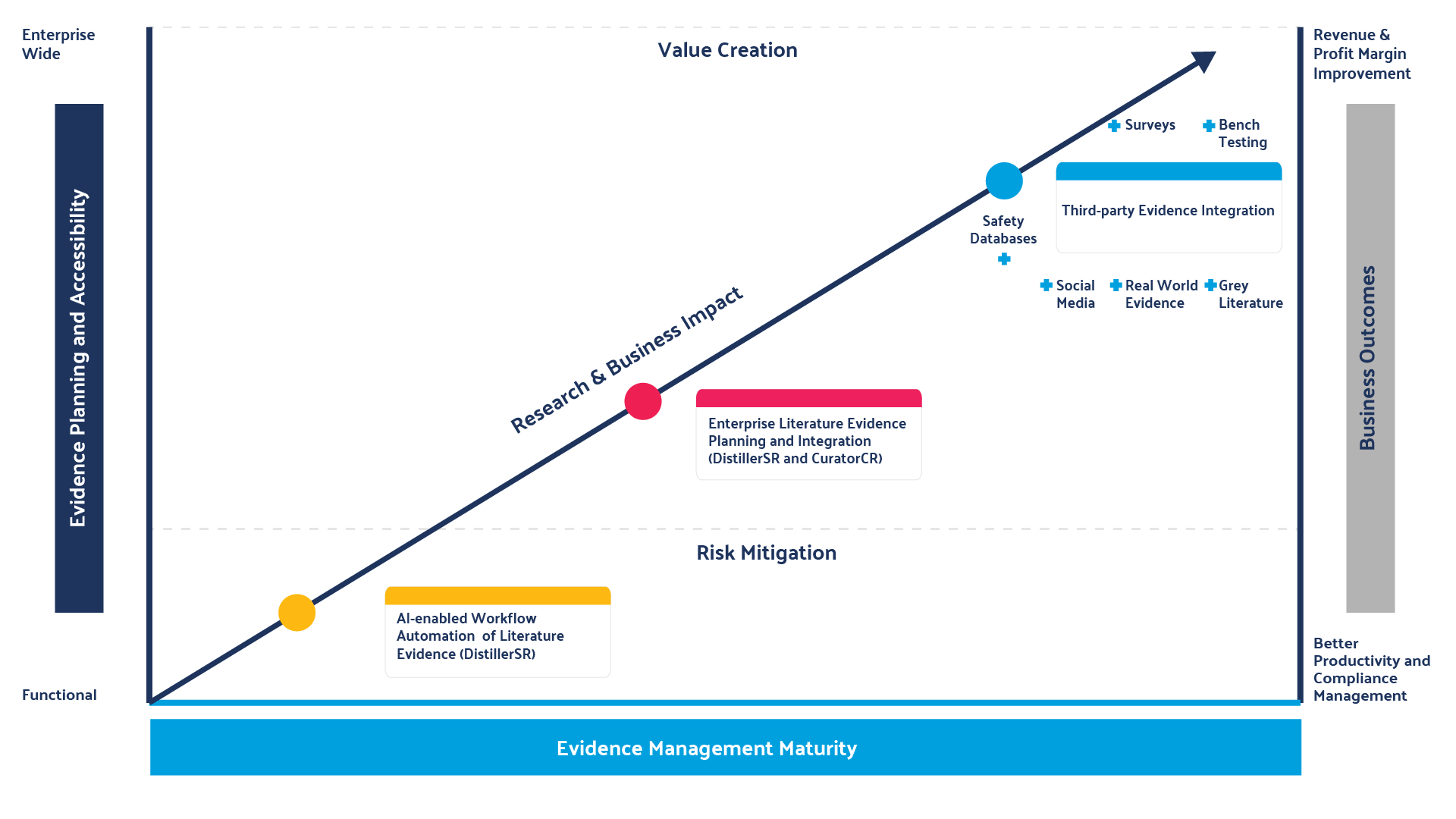
Here are the four stages:
1. Ad hoc – The processes for collecting, analyzing, reusing and accessing literature-based evidence within the organization are performed in an ad hoc manner, primarily at the function or project level, and are typically not applied across business areas. Evidence is restricted to project- or product-based silos, hampering collaboration and reuse. Process discipline is primarily reactive to unexpected events, and while improvements may be considered, they are not fed back into the organization at large.
2. AI-enabled Workflow Automation – There is awareness of the importance of managing evidence consistently at the functional level, with a set of standard processes and tools consciously designed to increase project efficiency and effectiveness, but adoption has not spread beyond a few functional areas. Some evidence-sharing occurs but the use of evidence-based decision-making is limited to the functional level, leaving business leaders in the dark. Process discipline may be fed back to the larger organization in an ad hoc manner.
3. Enterprise Literature Evidence Planning and Integration – Literature review evidence is treated as a competitive advantage, centrally collected and accessible to multiple functions across the organization, supporting the continuous curation, sharing, update, and reuse of data via API.
Data silos are rare or do not exist, fostering API data lake collaboration, efficiency, and risk reduction to regulatory approvals, product safety, and post-market surveillance activities. Process discipline is managed at the corporate level, with ongoing measurement, analysis, and improvement.
4. Third-party Evidence Integration – Literature-based evidence and other third-party data sources are integrated for developing timely and effective regulatory submissions. Data access is streamlined between internal and external groups via data lakes, enabling business leaders with a 360-degree view of all evidence-based activities. Process discipline is a critical corporate objective, encompassing most if not all, business functions.
How to Deploy a Successful Evidence Management Strategy
Implementing a centralized evidence management strategy aims to foster collaboration and facilitate data reuse across the organization to reduce rework and regulatory risks. Deploying a successful evidence management transformation requires a strategic approach that transforms the way an organization operates and follows four steps:
1. Define – This initial stage defines the objectives, scope, and goals of the evidence management strategy. This typically involves an assessment of current processes, technologies, and pain points across different departments and functions to identify areas for improvement, such as data management, research and development processes, and regulatory compliance. By engaging stakeholders at all levels, from C-level executives to operational staff, a foundation is set for aligning the company’s long-term goals with the needs and capabilities of the individual functional areas.
2. Pilot – The pilot stage selects a specific functional area within a company’s business unit to test and validate the proposed solution. This focused approach allows for controlled experimentation and learning to remediate any issues before moving to a larger-scale implementation. For example, a medical device manufacturer may choose to pilot DistillerSR on a small literature review project to identify any deficiencies in their traditional, Excel-based processes. This pilot should measure the impact of the change, such as improved process efficiency or fewer errors, and gather feedback from stakeholders to help refine the solution and champion its value to other teams.
3. Standardize – After the successful validation of the pilot project, the next step is to standardize the solution across relevant processes and functions. This stage integrates the new technology into existing workflows and proprietary tools, revises procedures, and provides training to employees. Standardization aligns the newly adopted technology with established best practices and regulatory requirements in various markets. Clear communication is essential to ensure all stakeholders understand the changes and benefits brought about by the change. Continuous monitoring, feedback collection, and iterative improvements are essential to fine-tune the implementation and maximize its effectiveness.
4. Scale – Once the solution is standardized and optimized successfully, it’s time to scale the transformation across the entire organization. This involves replicating the implementation in other relevant departments, functions, or geographic locations. Effective change management strategies are crucial to manage resistance, facilitate smooth adoption, and ensure a consistent user experience. As the transformation scales, it’s essential to maintain ongoing support, regular performance assessments, and adaptation to evolving needs. Feedback loops and data-driven insights from the initial pilot and standardized phases continue to inform refinements and enhancements, ensuring the long-term success and sustainability of the evidence management implementation.
Streamlining with DistillerSR Evidence Management Platform
DistillerSR automates the conduct and management of literature reviews allowing you to deliver better research faster, more accurately and cost-effectively. DistillerSR’s highly configurable, AI-enabled workflow streamlines the entire literature review lifecycle, allowing you to make more informed evidence-based health policy decisions, clinical practice guidelines, and regulatory submissions.
CuratorCR, a DistillerSR module, offers an integrated solution for healthcare organizations to enforce their evidence management processes. With CuratorCR, organizations can streamline literature and evidence curation, reuse previously collected data, and foster seamless collaboration across departments and external partners with permission-based access controls.
CuratorCR facilitates centralized evidence storage and retrieval, granting users access to a vast repository of references, full-texts, and evidence gathered from the literature. Its easy search capabilities and customizable filters ensure efficient retrieval of relevant information for various projects. Additionally, CuratorCR’s user-friendly interface and DistillerSR’s workflow automation features simplify the literature review process, minimizing manual effort and enhancing productivity.
A significant advantage of CuratorCR is its facilitation of data reuse. Through its centralized repository for evidence collection, CuratorCR enables researchers to utilize high-quality, peer-reviewed data, thereby preventing redundant research efforts. As a result, researchers can easily populate forms and tables with data previously collected by colleagues, a process streamlined by CuratorCR’s automatic identification of relevant information. This functionality accelerates the generation of critical insights, allowing researchers to make informed decisions more efficiently.
Moreover, CuratorCR facilitates knowledge sharing and collaboration by enabling organizations to securely share review data within teams or with external research consortia partners, such as universities and contract research organizations (CROs). This seamless collaboration ensures continuous access to up-to-date data, leading to quicker decision-making processes and improved operational efficiency.
Conclusion
The importance of optimizing literature reviews, managing evidence efficiently, and embracing data reuse for healthcare organizations cannot be overstated. The substantial operational and financial cost of siloed information and evidence collection practices are widely recognized by the industry. Nevertheless, there are significant barriers to changing corporate management practices and adopting common standards and ontologies in support of a governance model to manage evidence throughout an organization. By implementing a holistic approach to evidence, characterized by systematic collection, curation, and reuse of data, companies can break those barriers, fostering an environment of positive cross-functional collaboration, informed and strategic decision-making, which results in alignment across all levels of the organization.
Implementing a global evidence strategy cannot be seen as a mere operational enhancement but a strategic decision that will drive growth, accelerate regulatory compliance, and improve patient outcomes. As the healthcare industry continues to evolve, the ability to rapidly and accurately leverage a vast repository of data will become a competitive advantage. The journey is complex and requires cultivating a corporate culture that values data sharing and collaboration as well as implementing the right technology ecosystem.
References
- Seize the digital momentum: Measuring the return from pharmaceutical innovation 2022. Deloitte. January 2023.
https://www2.deloitte.com/us/en/pages/life-sciences-and-health-care/articles/measuring-return-from-pharmaceutical-innovation.html - Sertkaya A, DeVries R, Jessup A, Beleche T. Estimated cost of developing a therapeutic complex medical device in the US. JAMA Network Open. 2022;5(9):e2231609. doi:10.1001/jamanetworkopen.2022.31609
- Real-World Evidence. US Food and Drug Administration. 2023. https://www.fda.gov/science-research/science-and-research-special-topics/real-world-evidence
- Vantrappen C, Wirtz F. Making silos work for your organization. Harvard Business Review. November 01, 2021.
https://hbr.org/2021/11/making-silos-work-for-your-organization - Redmond TC. Bad data costs the U.S. $3 trillion per year. Harvard Business Review. September 22, 2016.
https://hbr.org/2016/09/bad-data-costs-the-u-s-3-trillion-per-year - Eastwood B. The case for building a data-sharing culture in your company. MIT Sloan. September 9, 2021.
https://www.gartner.com/smarterwithgartner/data-sharing-is-a-business-necessity-to-accelerate-digital-business
https://mitsloan.mit.edu/ideas-made-to-matter/case-building-a-data-sharing-culture-your-company - Amin P, Nam S, Pérez L, Smith J. Integrated evidence generation: A paradigm shift in biopharma. December 2, 2021.
https://www.mckinsey.com/industries/life-sciences/our-insights/integrated-evidence-generation-a-paradigm-shift-in-biopharma - Moore S. How to create a business case for data quality improvement. Gartner. June 19, 2018.
https://www.gartner.com/smarterwithgartner/how-to-create-a-business-case-for-data-quality-improvement