Two vital tenets of the systematic review process are transparency and reproducibility. I have long argued that both should apply at every stage of the review process, including title and abstract screening.
There are two schools of thought on title and abstract screening when it comes to the design of the screening instrument itself. One school advocates for a simple “voting” system, where two screeners use the screening criteria to include or exclude references at initial screening, without specifying the reasons for their choice. If the review was conducted using systematic review software, the screening form would have three options: “include”, “exclude”, and “can’t tell”.
The other school of thought asserts that not capturing reasons for exclusion violates the tenets of transparency and reproducibility; if no reason is recorded for the excluding a reference, how can an observer determine and/or validate how or why that decision was made? Not recording the reasons for your findings is, quite arguably, bad science.
As I have noted in previous blogs, not capturing reasons for exclusion also represents a missed opportunity. When you track the reasons for exclusion, you’re gathering valuable information on a study that may be useful in future reviews. It may also be useful should your inclusion criteria change in the future.
Consider the following example. In your first iteration of a review, you decide to include only RCTs and systematic reviews. Because of this, you screen out all of the cohort studies. At a later date, you decide to include cohort studies.
If you tracked reasons for exclusion the first time around, then you can reintroduce the cohort studies that were screened out, without having to re-screen them. If you used the voting system, you would have no way of knowing which references were excluded because they were cohort studies and, therefore, you would now have to re-screen every excluded reference.
Does it Take More Time to Capture Reasons for Exclusion?
The most common reason given for using the voting system over classification is that specifying reasons for exclusion takes too long. To get an objective measure of this, our team developed the following simple experiment.
Objective
To assess the impact on the quality and screening speed of two different title and abstract screening approaches. The first approach was to use screening forms that had simple Include, Exclude and Can’t Tell buttons. The second approach was to use three clearly defined inclusion criteria on the screening form to identify potentially relevant references.
Method
- We developed a systematic review protocol to assess the quality of systematic reviews published.
- A search was conducted in EMBASE and OVID to identify reviews that assess the quality of systematic reviews. After deduplication, the search returned 198 references for screening.
- Two discrete systematic review projects were created in DistillerSR systematic review software, and the search results were loaded into both.
- One project (Project A) was configured to use a simple screening form that asked if the reference should go on to full text screening (“Yes”, “No” and “Can’t Tell”). The second project (Project B) was configured to use a screening form that asked three specific screening questions.
- Both projects were configured to use two screeners. An inclusion result from either reviewer would promote the reference to the next level.
The screeners selected for both projects had equivalent domain expertise and experience in systematic reviews. - Both sets of screeners were provided with three criteria that must be met for a reference to be included. For Project B, these criteria were used as the inclusion/exclusion questions on the forms.
- Team B’s questions were arranged hierarchically on the form such that as soon as a reviewer answered “No” to a question, they could submit the form without completing the remaining questions.
- After title and abstract screening, results were reviewed by domain experts to assess how many, if any, references were excluded that should have been included.
- Time spent screening by each team was tracked by DistillerSR.
Project A Screening Form
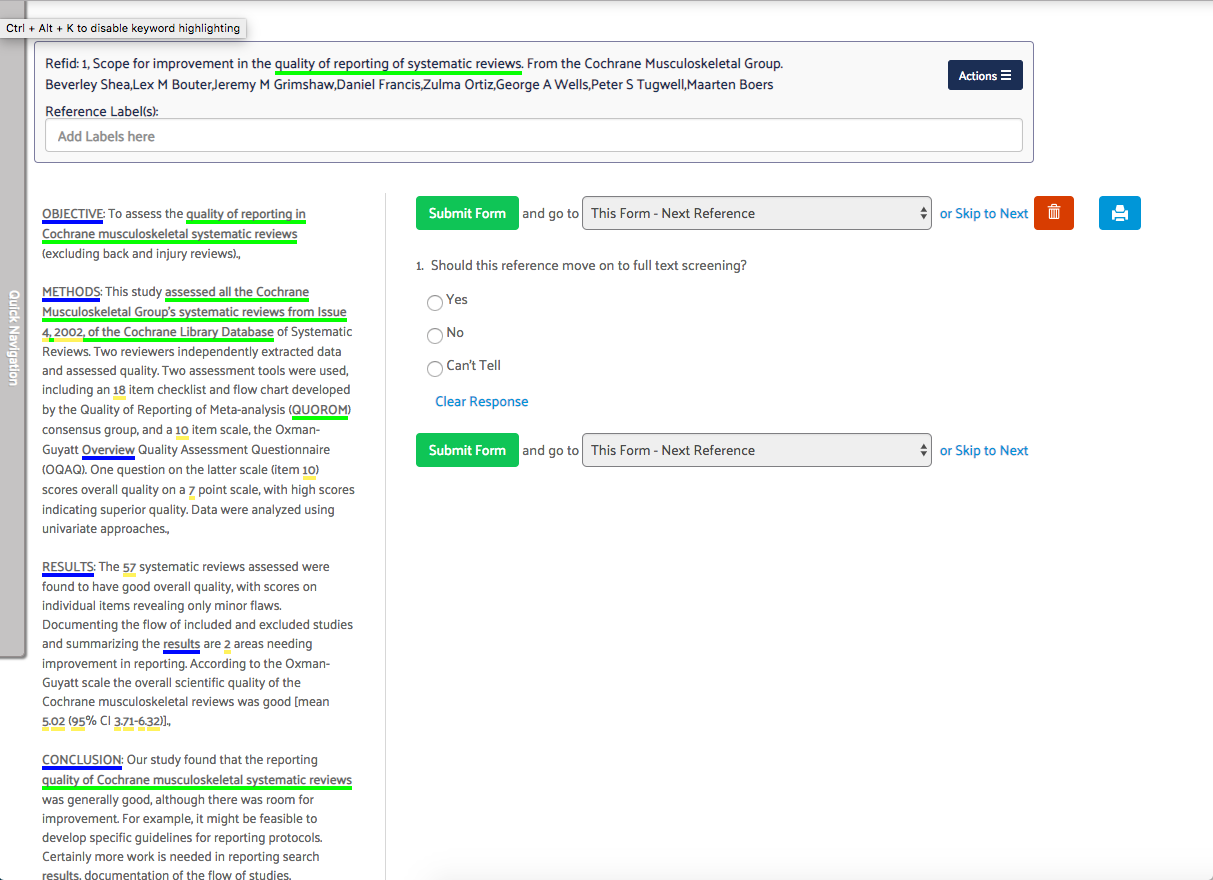
Project B Screening Form
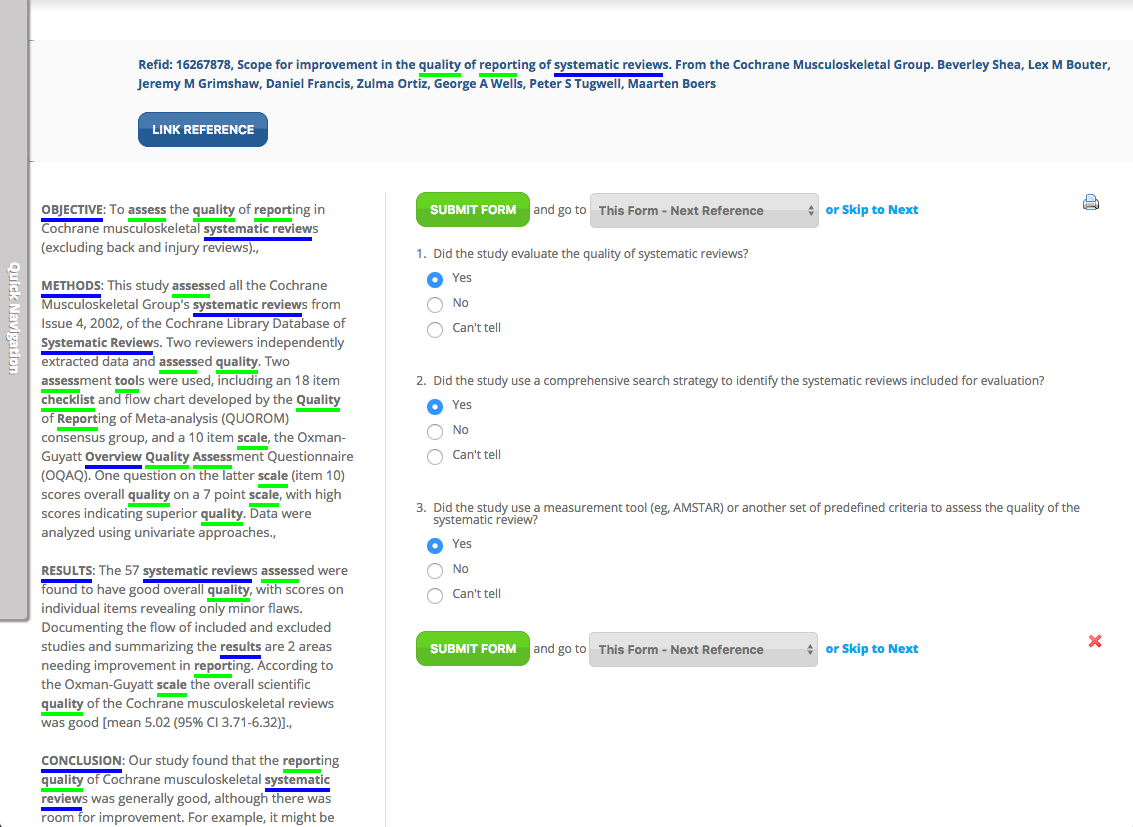
Results
Recall:
After analysis of excluded references, we determined that neither group had consensus exclude for references that should have been included. Recall was 100% for both teams.
Precision:
The team on Project A (yes/no/can’t tell) achieved consensus exclude on 139 (70%) references at title and abstract screening.
The team on Project B (reasons for exclusion) achieved consensus exclude on 157 (79%) references at title and abstract screening.
Team B achieved higher precision than Team A, sending 18 fewer references to the next stage of screening.
Team A’s median time per reference screened: 20 seconds.
Team B’s median time per reference screened: 14.5 seconds.
Median time per reference spent by Team A’s faster screener: 10 seconds
Median time per reference spent by Team B’s faster screener: 11 seconds
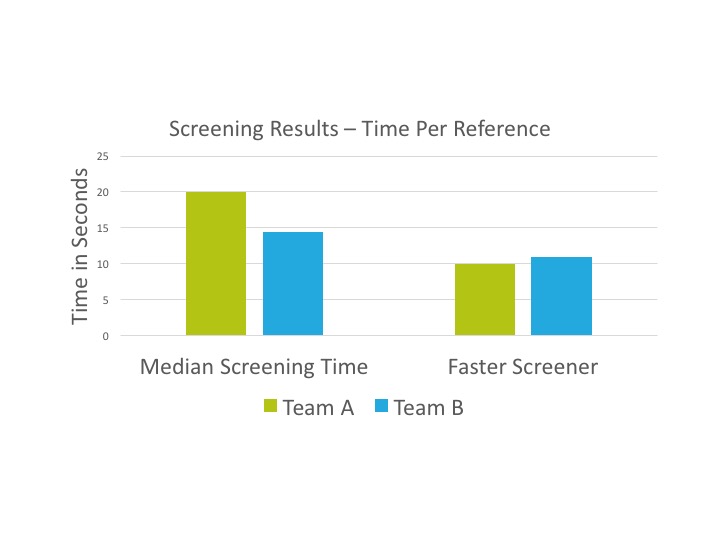
Reviewer Calibration:
Team A recorded 37 disagreements between reviewers in the Yes/No/Can’t Tell screening question.
Team B recorded 16 disagreements between reviewers across all three screening questions.
Observations
Even though the skills and experience of both teams were roughly equivalent, the team that recorded reasons for exclusion (Team B) had a median time per reference that was faster that the team using the simple voting strategy. Team A’s fastest screener had a median screening time that was 1 second faster than Team B’s fastest screener.
In a post-screening interview, Team A noted that they would frequently refer back to the screening criteria when voting and this, they felt, added time to the screening process.
Team A screeners selected “Can’t tell” 34 times, compared with 12 times for Team B screeners.
Conclusions
Although this was a single experiment with relatively small numbers, the results demonstrated that capturing meaningful reasons for exclusion did not add significant time to the screening process and that, when we measured the fastest screener, the two processes were roughly equivalent in time spent.
Furthermore, the group capturing reasons for exclusion was more precise, reducing the amount of time and resources spent at the next level of screening. Thus, from an overall project perspective, capturing reasons for exclusion reduced the overall time to complete the project.
In the case of the references excluded by Team B, a clear record of why each reference was excluded now exists. This data can be used in peer review, regulatory review, review updates or in future projects that use these references.
Let’s Make N Bigger
Were you surprised at these results?
This small experiment was a first step, and more data is always better. If you have a two person team with about 2 hours to spare and you would like to see how you compare to our test teams, please contact us. We’ll set you up on an identical test project, measure your screening process, add those values to our dataset and share the results with you.
Based on past experience working with many different review groups, I wasn’t surprised at all to see that recording reasons for exclusion doesn’t necessarily take more time than a simple yes or no vote. I believe that presenting reviewers with inclusion/exclusion criteria right on the screening form keeps them on track and focused, which may improve the speed and accuracy of their screening.
I also believe firmly that capturing reasons for exclusion is critical to maintaining transparency in the systematic review process. It may have been an additional administrative burden in the past, but with today’s software tools that make it easy to track and manage every part of the review process, there is no reason not to do it.